DOES TRANSFORMATION HAVE TO BE TRANSFORMATIONAL?
TGG Senior Consultant, Danny Quarrell recently supported a large client partner with a key Transformation initiative. Below Danny shares details and insights on how a mix of enablement projects alongside game changing Transformational projects are key to a healthy Actuarial Transformation Program.
Does transformation have to be transformational?
The biggest ongoing question in my first actuarial transformation program engagement seems like a trick question. Of course, it’s supposed to be transformational! It’s literally called The Actuarial Transformation Program.
Well not so fast my friend!
Rather than looking at an Actuarial Transformation (AT) program as a group of transformational projects, it should be viewed as a spectrum of projects that have a range of transformational outcomes. The end goal is transformation, of course, but each project contributes to that goal in different ways.
The projects that have the least transformative impact can often be the most overlooked and under prioritized projects in the program. Yet these types of projects are worthwhile (and even required) activities that create an opportunity for transformation where there previously wasn’t one and work to keep your community engaged. We refer to these types of projects as transformation enablers.
Transformation enabler projects will typically share these characteristics.
1. They free up time
2. They reduce risk
3. They are an iterative step on a path to a transformational solution
4. They keep the actuarial community engaged in the program
5. They build trust with the broader organization that leadership is actually committed to seeing a transformation through to actual transformative results
Before we get too far into our discussion, let’s be clear on what an example of a transformational project would be. Let’s say you have multiple groups of actuaries that rely on the same modeling calculation platform, but each team prepares their data (inforce data, assumption data, etc.) in their own way for ingestion into the platform and they each have different ways of storing outputs. Maybe one group relies heavily on Excel, another uses R and SQL, and maybe a third group uses MS Access. A transformational project might look like:
Building an enterprise-wide application that would allow each team to manage their inforce data, set assumptions and initiate model runs through a common interface and process. The application would also manage model results and provide robust tools for governance, controls, and auditing.
I think most people would agree that kind of project would be very transformational within an actuarial organization. It would reduce the time needed to prepare data and create the ability to produce multiple model runs in a shorter time frame, reduce risk, reduce ramp up time for actuaries rotating between teams, and streamline the governance process since all models would use the same processes.
Game Changer!
These game changing types of projects are the stuff dreams are made of; they’re also fraught with risk! They take years to implement, have high costs, require incredible levels of trust and engagement from your subject matter experts, and they can sink your program when they go wrong.
These risks are exactly the types of risks transformation enablers are meant to address. Let’s drill down a bit.
· Major transformational projects take years to implement and can easily lose momentum or fail to win the support of the people they are meant to help
Enabler projects can provide shorter term wins, keep the actuarial community excited about the future and help them stay engaged with the program
· Not every team is ready to be transformed, some people are just trying to keep from sinking!
These teams need relief, not transformation. They don’t have the time to stay engaged with the solution that you want to build and release 18 months from now. Give them the relief they need, then engage them on transformation
· Legacy processes are often fragile and have high risk. Process owners don’t want to exacerbate risk with change
Process owners for legacy processes know where their processes are fragile and where the risk lies. Iterative solutions can provide targeted solutions to these key areas. Lowering risk and the stress of owning the process.
· Legacy processes can benefit from being improved iteratively, and stakeholders are more likely to provide valuable feedback when given multiple opportunities via iterative solutions being presented
When you present iterative solutions that start by alleviating key pain points the subject matter experts and key stakeholders are more willing to engage on further improvements and accept greater change to the processes they own. Rather than asking for trust when you promise a big benefit down the road, earn trust by presenting small benefits now.
The actual enablement projects will vary from program to program and team to team, but the characteristics will remain the same.
1. They free up time
2. They reduce risk
3. They are an iterative step on a path to a transformational solution
4. They keep the community engaged in the program
5. They build trust with the broader organization that the leadership is actually committed to seeing a transformation through to actual transformative results.
New organizational initiatives pop up every quarter but the surest way to achieve collective buy-in is to make the real work visible, recognized, and a focused priority. A mix of enablement projects alongside your game changing Transformational projects are key to a healthy Actuarial Transformation Program.
At The Gunter Group our experienced team has decades of experience leading transformational initiatives for client partners. Our versatile team knows change doesn’t happen overnight and are ready to help your organization make transformational progress and achieve transformational results.
DON’T LET TECH DEBT STAND IN THE WAY OF DATA-DRIVEN INSIGHT
Technical debt can put organizations in a headlock, both in the short and long term. Almost nothing casts a bigger, scarier shadow for decision makers — the perception of the time and cost necessary to overcome tech debt looms large, keeping entire companies frozen in fear.
This burden makes it difficult to efficiently extract insights from data. It’s a recipe for stifled growth.
We’re here with a message of hope: you don’t have to dive into a resource-intensive, five-year transformation project in order to manage your technical debt and realize your data potential. You have other options, and it all comes down to prioritization.
What is tech debt?
Technical debt is often defined as the cost incurred when you repeatedly choose short-term solutions rather than doing the (larger, more expensive) work of tackling the big-picture causes of your problems.
But let’s look at the issue through a different lens: what is the nature of technical debt?
Because new solutions are built and deployed every day, all organizations incur tech debt, to some degree, with every system and process implementation decision they make. Even if you implement a new, innovative solution today, there will be a better one available tomorrow. In this scenario, you will still incur tech debt — just less than an organization that makes no updates.
Too many organizations think of tech debt as a problem that can be permanently solved. In reality, it’s a constant that’s renewed continuously by change and growth, and trying to “solve” it completely is a futile pursuit.
While you can’t make tech debt vanish into thin air, you can certainly make it more manageable. If you focus on managing its impacts in an ongoing way, you can deflate its looming, monstrous reputation and get to work on making meaningful improvements in the here and now.
Is tech debt destroying your data-driven dreams?
Analytics bottlenecks are a common issue related to tech debt. Silos slow down the analytics process; if only one person knows where a spreadsheet is and how to extract meaningful data from it, they become the bottleneck.
With each short-term fix and siloed process, data becomes harder to manage, access, and analyze. In turn, drawing insights from that data requires more time and effort, the insights become less timely and less reliable, and informed decision making becomes more challenging.
In other words, tech debt has a way of draining value from data — and the longer you let that debt accrue, the more value you’re losing. Using a prioritized approach to managing tech debt can help you cover more ground right out of the gate, so you don’t lose any more value than you have to.
One way to apply this prioritized approach is with backlog grooming, the periodic process of reviewing and prioritizing backlog tasks (and removing unnecessary or outdated tasks).
How do you prioritize what areas to address?
There is a lot of information available on how to tackle tech debt. Unfortunately, most of it is theoretical. While the abstract stuff can be valuable, if you’re looking for a practical way forward, you need to bring your considerations back down to earth and fold in the business perspective to create a technical debt prioritization plan.
You probably have a lot of tools at your disposal, internally and externally, and resources to leverage. Take a look at what you need to have happen — not theoretically (e.g. eliminating all technical debt by some point in the future) — but actually.
For example, The Gunter Group recently worked with a retail automotive company that was struggling with data debt. It was impacting every area of their business, including employee satisfaction, customer satisfaction, and profit margin. They needed a new approach, but with such a vast problem, it was difficult for them to know where to start.
We worked with multiple teams within the company, including manufacturing, HR, marketing, and technology innovation to create a prioritization plan. High priority initiatives included redefining company-wide KPIs, designing and implementing machine learning algorithms, and improving data literacy across departments.
Though they still have a long way to go on their data maturity journey, this company was able to start making changes where it mattered most, rather than remaining paralyzed by the challenge ahead.
How we work with clients to tackle tech debt
Remediating data-related tech debt requires far more than just technical skills — it requires asking the right questions, gaining a holistic understanding of your organization’s business goals (as well as how they may vary across different departments), and creating a dialogue to explore possible solutions.
Each of these components requires a tremendous amount of time, which internal teams rarely have. In most cases, managing ongoing operational struggles takes priority over transformation, and team members don’t have the capacity to focus all their energy on addressing tech debt. Meanwhile, recruiting new team members is a time-consuming, resource-intensive process, and thanks to the tech talent shortage, it’s more challenging than ever.
Turning to outside help can get the data transformation ball rolling without overwhelming internal teams or opening a can of recruitment worms.
At The Gunter Group, we leverage a multidisciplinary approach (technology, people, strategy, and execution) that enables us to see the long-term big picture while solving the highest-priority problems in the short term.
Combined with our extensive technical capabilities, this approach allows our clients to chip away at their technical debt and reclaim the value of their data as quickly as possible — without the burden of hiring a new team.
Conclusion
Think about a meaningful, specific problem you’re facing right now that’s rooted in technical debt, and what you would be able to accomplish if this problem was being managed proactively.
If you set your sights on eliminating tech debt across your entire organization, you’ll likely get caught up in a complex tangle of issues — and that one major problem that’s holding you back now will still be holding you back in six months.
To accelerate your progress, identify your most pressing issues, and reach out to expert help if you need it. With the right strategy and the right partner, you can mitigate tech debt and use your data to its fullest potential.
Is technical debt slowing you down? Discover how to improve your data infrastructure and decision making with workshops hosted by The Gunter Group.
WHY ISN’T YOUR DATA TRANSFORMATION PROGRAM TRANSFORMING ANYTHING?
It’s a familiar tale — you’ve embarked on a journey to transform the way your company uses data, but all you have to show for it is a lot of very ambitious documentation and hundreds of progress update meetings where time feels like it’s standing still.
Why isn’t your in-progress data transformation program producing tangible results? Here are three common reasons why your efforts might be failing to deliver:
1. Your approach isn’t incremental.
We recently wrote about data maturity models and how they can actually hinder data transformation if you don’t apply them correctly. To summarize, organizations tend to focus too much on the brass ring (reaching some proverbial data maturity nirvana) and not enough on the details (getting more value from data along the way).
Often, the goal is a total overhaul of how data is used from the top to bottom of the business, delivering unimaginable value to everyone, everywhere — an ambitious transformation that will take years to achieve. Even if you’ve had a few updates and helpful process changes along the way, most of the value remains locked behind the “project completion” door.
What does that mean for those who are trying to get some incremental improvement and use data more effectively in the near term? It means they have to just sit tight and wait for the big reveal — otherwise, they’ll be doing work that’s not aligned with the broader strategy.
It’s important to consider the folks in your organization who use data to complete everyday tasks, and how you can make their jobs easier, not harder, during the data transformation process.
2. Technical debt is handcuffing you.
Individual innovation can be a huge asset for an organization. You want people to take initiative, solve problems, and make it happen. But when it comes to managing data, all that individual problem solving can add up to huge technical debt, and make organization-wide data transformation efforts feel insurmountable.
When everyone has their own unique, siloed quick fixes and band-aid solutions, there’s no more unifying structure left to transform — so where do you even start? Think of the chaos you’d unleash by pulling at even one loose thread; mission-critical systems that were crudely patched together would come tumbling down.
As an additional challenge, there will always be responsibilities and processes that can’t be switched off while things are fine tuned. For example, if you’re using your existing data systems to calculate monthly commissions, that presents a huge barrier to making any meaningful changes — you can’t stop paying out commissions until changes are implemented, and it would be risky to just cross your fingers and hope that brand new systems produce accurate results in time for you to cut checks.
Getting out from under technical debt isn’t impossible — it just requires a lot of effort. It’s also infinitely easier to achieve with the right combination of planning, skill, and resources, which is why many companies partner with firms that specialize in tackling technical debt.
It’s also important to remember that tech debt isn’t something you can escape completely, but a challenge that needs to be continually addressed. Good solution design assumes that today’s new solution is tomorrow’s tech debt, everything is eventually deprecated, and all solutions incur maintenance and upkeep. Finding the balance between standardization and innovation is important, as too much of either can be stifling.
3. You don’t have the right people.
Pulling off a successful data transformation project takes a lot of skill sets (strategic planning, project management, change management, ETL development, data science, etc.), and it’s highly unlikely that you’re going to find a single person who can do them all well. It’s also unrealistic to expect your current staff to sustain day-to-day operations and magically find the time to make changes. In 99% of cases, you’ll need outside help.
Trying to build an in-house team is difficult, especially in the midst of a data analytics talent shortage. Planning and recruiting for a team can take anywhere from six months to a year, plus the time it takes to get everyone up and running. If your organizational culture (or even just a particular decision maker) is change-resistant, this process can take even longer — and feel like pulling teeth.
Technical leaders often have to spend a large amount of time jockeying for resources and pitching projects. You may have to argue and advocate for three months to hire one person that was needed to solve a problem three months ago. By the time you get a decision, you’re going through the same cycle with a different problem.
It’s easy to see how years can go by with little to no progress.
This is another situation where it can be helpful to work with a data transformation partner, which gives you access to highly skilled, experienced people, without the pressure or long timeline of trying to build an in-house team.
Conclusion
Our general advice for data transformation can be summed up in two words: don’t wait. Every day you remain stalled out, you acquire more technical debt, and your problems get more complex. You lose more business, you gain less on your competition, and your company loses value.
If you need to see real outcomes from a data transformation project, focus on incremental improvements, and find a partner who can help you overcome the challenges we’ve outlined here.
Above all, remember that data transformation isn’t a “project” that begins and ends. It’s a decision to become a data-oriented organization — and it takes continuous effort and agility.
Not seeing results from your data transformation initiatives? Discover how to improve your data infrastructure and decision making during our upcoming Data Maturity workshop on December 15th at 11:30am Pacific Time.
CHANGE MANAGEMENT:
THE PAST, PRESENT, AND TGG
People are hardwired to be cautious of change. However, in today’s rapidly-evolving business climate, organizations must find ways to support employees through inevitable changes. From operational restructuring to software implementations and everything in between, the field (and process) of change management is complex.
With the numerous methods, techniques, and approaches to change management how does an organization identify and pinpoint the best path forward?
It will help to briefly travel back in time to understand the roots of the formalized practices that flourish today and break down three foundational pillars of change management that organizations can utilize today.
The Migration From Psychology To Business
Change management has roots in the study of human behavior. The intellectual beginnings trace to the early 1900’s, into the work of the anthropologist Arnold van Gennep. When looking at rites of passage in different cultures, Gennep began to notice common behaviors.
Even though he was looking at a variety of different cultures, he noticed that there were three overall states that ran common in the experience of social change. The first state was a “pre-liminal” stage, where the coming change was acknowledged and prepared for by the community.
The middle state was the “liminal” stage, which he defined as a threshold of ambiguity and disorientation. Change managers everywhere will chuckle at the accuracy of adjectives like “ambiguity” and “disorientation” when describing the liminality of change.
The final state in a rite of passage was “post-liminal,” where the transition in status was recognized and normalized in the community. Across cultures and belief systems, Gennep was able to identify these common movements in the human experience of change.
By the mid-20th century, when psychology began to blossom into a robust and complex discipline of study, Gennep’s three states gained popularity. In the 1940’s, Kurt Lewin became a pioneer in social and organizational psychology by turning his attention to understanding change. Lewin borrowed from Gennep’s structure and described a three step process for change: unfreezing, changing, and refreezing.
There was some academic buzz from several sources in the years following Lewin’s work, but no substantial leap from psychology to business had yet been made. This changed in 1982, when a consultant at McKinsey named Julien Phillips published an article in the journal Human Resource Management.
In his article, Phillips introduced a model for organizational change management specifically designed with businesses in mind. His model defines four steps that were intended to build momentum for change within an organization: creating a sense of concern, developing a specific commitment to change, pushing for major change, and reinforcing the new course of action.
In the years following, change management took off. Books were published; articles became more frequent; new models were advanced. Businesses were in need of assistance with change, and consultants pursued thought leadership that would help address this need and grow their business. Peters, Waterman, Kotter, and dozens more developed robust philosophies and methods for change, and organizations bought in and helped the field to grow.
Today, there are as many models for change management as there are consulting organizations. Looking for a 4 step process? Try PDCA. Interested in 5 steps? Try ADKAR. How about a 6 step approach? Try Pulse. Need more? Try Kotter’s 8 Steps, or Prosci’s 9 Steps. There are symposiums and communities of practice such as Prosci and ACMP; and naturally a veritable cornucopia of certifications abound. Change management is so saturated with models and approaches that some even try to push “beyond change management.”
100 Frameworks, 1 Idea
The Gunter Group does not subscribe to any one framework. Our clients are too unique for a single set of steps to be the answer. We proudly proclaim ourselves to be “methodologically agnostic,” much more interested in understanding the organization than blindly peddling a process that fails to fit the people it is meant to help.
That is not to say that we don’t know the methods. Our consultants have expertise in Prosci and ADKAR; and we have a number of tangentially relevant certifications (Six Sigma, SAFe, PMP, and HCD to name a few); we attend local ACMP events. We do not, however, learn a method to become disciples. Rather, we expose ourselves to frameworks and study methodological vocabulary to leverage those aspects of the frameworks that might be helpful for our work. Our clients appreciate a tailored approach that is grounded in the best practices of 100 frameworks.
This approach to consulting reveals something obvious: all change management methods are basically the same. Decades of scholarship and praxis have not changed the core phases of change, and wisdom that dates back a century still lies at the heart of responsible change. There are 3 basic phases in change (before, during, and after), and every change management framework simply iterates on the approach taken within those three phases.
So what runs common throughout all change management? What activities should you keep in mind as you tailor the process to your specific organization? We’ll run through the basics below.
Step 1: Pre-Change
Change is coming. Perhaps it is a changing regulation, a new technology, an upcoming merger, or a poor quarterly report; whatever the reason, you see change on the horizon and understand that you will need to prepare for it. Though the various frameworks approach preparing for change differently, three key activities take place during pre-change: analysis, planning, and influencing.
Analysis comes first. Before you can plan for change, you have to understand the people and processes that will be impacted. Who will be your champions, sponsors, and resistors? Helpful tools for this phase include stakeholder matrices, current state and future state process maps, and change impact assessments. The change manager must also understand the change itself. Without a powerful grasp on the “why” that is driving the change, planning and execution will fall short.
Planning comes next. Change management occurs somewhere between the intersection of strategy, people, and execution, and planning is the bridge that brings these three elements into alignment. This includes planning for the change itself, communication that will accompany the change, and the training that will make the change possible.
Influencing should follow. ADKAR describes this as fostering awareness and desire. Prosci speaks of sponsors and champions. Other schools of thought suggest using concepts like vision or need. We have found that a cocktail of all these approaches is usually the best way forward.
Step 2: Change
You’ve spent time interviewing stakeholders, mapping processes, and planning training sessions; now it’s time to introduce the change. This is messy, confusing, and difficult for the people impacted so change managers often rely most heavily on a methodology in this phase. However, mid-change is where a generalist approach could be most advantageous, adapting to the ongoing needs of the situation. There are four activities that always occur in any well-managed change approach: communication, training, changing, and reinforcement.
The most important activity surrounding change is communication. This is where you lean heavily on the results of your analyses. You know who needs communication, what they need to hear, and how it will affect their work flow. Armed with this information, you can plan accordingly, communicating the upcoming movements to the right people, early and often.
Another essential activity is training. This often goes hand-in-hand with communication, and is best when designed from the viewpoint of those impacted. Recent developments of tools such as Human Centered Design help maximize the value of training.
At a certain point, the change will happen. Kotter recommends an approach of small-slicing the change to create short term wins, but often the change manager is not the one driving the project timeline. When it comes to go-lives, change managers serve a thousand roles. They become SME’s for elements of the change impact; they attempt to remove obstacles from stakeholders; they act as cheerleaders or bulldogs, whatever is called for in the moment.
As change occurs, another important activity is reinforcement. A big part of this is engaging program or organizational-wide leadership to enforce the change. This activity truly begins in pre-change and extends through the end of post-change, but it becomes extremely important in the midst of the change. There are approaches coming out of organizational psychology that can be helpful here, such as Vroom’s Theory of Motivation, McClellan’s Theory of Three Needs, or McGregor’s Theory X and Theory Y.
Step 3: Post-Change
Follow-through is a must. As Gennep would say: the new status must be confirmed and the change must be reincorporated as the new norm. This is done a little differently in each framework, but necessary activities include reinforcement and reanalysis.
As said above, reinforcement is heavily featured in post-change activities. The goal is longevity, driving the change through ongoing champions and dwindling resistance. Success is celebrated, momentum is reinforced, and improvements are consolidated. Through these activities, the new order is anchored in behavior.
One often-forgotten activity that takes place after the change occurs is reanalysis. Throughout this whole process, you’ve generated a mountain of information, from stakeholder input to process metrics. Current-state assessments performed before, during, and after the change are a great way to analyze that information, evaluating the effect of the change.
Change management is the study of human behavior. Change is inevitably difficult for humans, yet change is unavoidable. As professionals in change management, we bring a people-centered approach to our work. We partner with clients to ensure employees and stakeholders understand, support, and adopt the desired change and it starts with a very critical element: listening. We view our clients’ change as if it was our change, and their people, as if they were our people, with a foundation of respect.
WHAT MAKES FOR AN IMPACTFUL CHANGE LEADER?
Impactful change leaders understand not only the holistic process of change but also the simple process of change, disruption, adaptation, and confirmation. They know the tools and the steps to help guide them in executing that change.
Great change leaders think systematically. They’re fluent in the interwoven nature of change. For example, the reliance on, and the potential impact to, a long-term strategy: the new business opportunities that are presented, the operational impacts, the cost impacts and implications, and the efficiencies that could be gained from a change.
The best change leaders are those who recognize that there’s an absolute, inextricable, undeniable criticality of engaging their people and their teams in bringing about change. No process, no strategy, no business model, no ROI, no calculations will matter if there’s an absence of engagement on the team.
When faced with challenging situations effective change leaders have the ability to:
1. Respond in a timely manner to a market force or operational inefficiency.
2. Understand that change is a necessary process, and that it will happen again.
3. Learn from the challenges in front of their organization and learn from the change process they undertake.
4. Challenge the status quo and ask strategic unbiased questions with a focus on improvement and solutions.
5. See how change can and will impact their organization at an enterprise level.
6. Explore new processes and tools to support and scale change and improvement.
7. Communicate clearly and effectively to their team so that team members know the “why” behind the change and understand how their role fits into both the process and the outcome.
Large-scale change takes place across all industries with some of it intentional, and some of it unexpected. Regardless of the forcing mechanism, effective change leaders find ways to engage teams at a more human level, and in doing so choose engagement over exclusion and as a result prioritize organizational health and success.
As professionals in change management, we bring a people-centered approach to our work. We partner with clients to ensure employees and stakeholders understand, support, and adopt the desired change and it starts with a very critical element: listening. We view our clients’ change as if it was our change, and their people, as if they were our people, with a foundation of respect.
A QUICK AND DIRTY GUIDE
TO DATA MATURITY
You’re probably familiar with the concept of data maturity — a measurement of how well an organization uses its data — and the maturity models that go along with it.
Understanding your current level of data maturity is the first step toward improving it. But the way you interpret and apply data maturity models might actually be hindering your success.
It’s tempting to look at a maturity model as a straightforward tiered system — your organization exists in one category, and your goal is to move up to the most mature category. But particularly for larger organizations, things aren’t so clear-cut. It’s common for different departments or business units to be at different maturity levels. Additionally, too many organizations get caught up in the long-term goal of data maturity and miss out on opportunities to create value at every maturity level.
In this article, we’ll go over the four categories we use to assess data maturity, give examples of challenges that arise in each category, and offer recommendations for driving more value at that level.
Before we get started, here’s a summary of each category for context:
Note: As you consider where you fall, it’s important to be realistic — think about where you are right now, not where you hope to be after completing a particular project. It’s also helpful to remember that it’s rare for an entire organization to exist within a single category. In most cases, different departments and teams will have different maturity levels.
Siloed
What it looks like:
Point-in-time data is manually exported from various applications and pulled into spreadsheets on an ad hoc basis. Team members compile and analyze data in their own individual workspaces (e.g. a spreadsheet only you have access to, or a platform that’s only used by one particular team).
Finding data requires some exploration — team members are not always sure where to look or who to ask for the data they need. They often end up emailing or asking around until they find someone who has what they’re looking for.
Example of a challenge:
You’re planning to hire people for a new team, and want to use a data-driven recruiting approach. You need access to key data about past recruiting efforts, like cost per hire, time to fill, recruiting yield ratios, and first-year attrition, so you send an email to HR requesting the information. HR then needs to take the time to locate and compile the data into a spreadsheet and send it to you.
Because you don’t have immediate access to the data you need, the recruiting process is already slowed down, and it will take longer to get your new team filled.
How to drive more value:
The importance of business process management can’t be overstated. For a process that will undoubtedly need to be repeated in the future (like looking at recruiting data), it’s important to start out with a deep, thorough understanding of the process itself. The first step to creating a repeatable environment is understanding what you want to repeat and why — then you can work on implementing more efficient processes.
Standardized
What it looks like:
Your organization has standard, scheduled operational reporting. You don’t have to go on an expedition every time you need data, because you know that you can find it in the most recent report (however, you’re still manually pulling data from that report). Even if you’re creating useful insights, they tend to stay with you — they’re not shared with other teams or business units.
Example of a challenge:
Each week, everyone in your department receives an email with an automated report that covers POS data. Most of the time, you don’t find anything in the report that’s actionable for you, so you sometimes don’t even open it.
When you do read it, you have to comb through tons of data to find anything relevant and manually extract it, which is time-consuming and inefficient. You complete analyses that are important to you within your own workspace, and the results aren’t usually shared outside of your immediate department.
How to drive more value:
Once you know where to find your data, determine what makes it actionable and relevant — and who needs to see it. As a starting point, ask yourself the following questions:
- What decisions do I need to make, and how can this data inform those decisions?
- What’s the ideal way for me to receive and view this data?
- Who else would benefit from seeing this data?
- How can data be efficiently shared across departments so everyone has access to what they need?
Enterprised
What it looks like:
When a particular threshold that’s relevant to your job is met, you automatically receive the relevant information. Rather than getting a standardized report that may or may not contain information you care about, you get notified only when there is data pertinent to a decision you need to make or action you need to take — whether that data is related to a budget milestone, warehouse stock, page views, or something else.
Sources of data are aggregated, so when you need insights, you don’t have to manually combine and analyze data. However, the reporting is still fundamentally historical and often comes too late to help you make informed decisions.
Example of a challenge:
You’re responsible for mapping out an upcoming seasonal campaign and need sales data to inform your plan. Each week, you receive an automatic report containing data that helps you shape the campaign, but you have no way of looking forward in time — you’re stuck with a decision-making process that is reactive, not proactive.
How to drive more value:
With the right timing, data can be leveraged to achieve better outcomes (and predict possible future outcomes). Don’t just think about what data you need and where to find it — think about when it will have the biggest impact on decision-making, and how it can help you course correct before things get off track.
Actualized
What it looks like:
Your data is set up to model and predict future outcomes, perhaps using AI tools like predictive analytics and decision algorithms. Analysts and data scientists are an integral part of the business vision, and insights are created by the company (not requested by specific business leaders).
For many companies in this realm, the data model is inextricably linked with the product or service they provide. For example, platforms like Netflix and Spotify are rooted in predictive data analytics and the ability to make personalized recommendations to customers.
Very few companies reach this level of data maturity — and the reality is, not every company needs to. It takes continuous investment to maintain an Actualized data system and depending on the products and/or services you provide, it might not deliver enough ROI to justify spending the resources and effort.
Example of a challenge:
Your organization is experiencing a slight decrease in customer retention. You already have access to real-time data and analytics that help you understand the problem, but you want to leverage that data in new ways in order to make more informed decisions.
How to drive more value:
Being an Actualized organization doesn’t mean you’ve crossed the data maturity finish line — in fact, there is no finish line.
Only the most advanced companies make it to this level, which means competition is stiff. And considering the incredibly fast pace of data technology, companies that don’t continuously innovate will be left behind.
At this stage, optimization is key. Consider how data can better drive decisions and open up new opportunities for your organization. In the case of the challenge above, building new algorithms for churn models could help guide decision-making and reveal more actionable data.
Conclusion
Identifying your current data maturity level and setting goals for improvement is all well and good, but without taking steps to get more value from your data at your current level, your long-term progress may stall out.
Rather than thinking of data maturity models as rigid paths with set destinations, use them as way-finding tools. Once you understand where you are, you can move forward — no matter what “forward” looks like for your organization.
Regardless of your maturity level, we can help you get more value from your data. Discover how to improve your data infrastructure and decision-making with our Data Maturity Assessment.
SURVEYING THE DATA LANDSCAPE IN 2022
In the past, data wasn’t necessarily important to every person within a company. It was used primarily by analysts, accountants, and other specialists.
But in 2022, companies are learning that becoming a data-driven organization means incorporating data into every aspect of their business — from talent management to customer engagement and beyond — and continuously optimizing how they use data with new innovations and process improvements.
What does being data-driven look like in action? Here’s an example: a west coast retail automotive company employing over 7,000 people across 9 states came to us with the goal of implementing a mixture of data science and machine learning to identify, implement, and improve safety, employee satisfaction, customer satisfaction, and profit margin. The client asked us to work with multiple teams within manufacturing, HR, marketing, and technology innovation to build out the desired capability.
To help this company reach their goals, we provided high-level strategic insight for new initiatives, built out proof of concepts, made recommendations for innovative methodologies, designed machine learning algorithms, helped them redefine company-wide KPIs, and trained their staff on new processes.
As a result, executives are better able to make key strategic decisions and further company goals based on data-driven insights, and the entire organization’s data literacy has improved.
A shifting mindset
A few years ago, the goal for many companies was “fixing” their data processes (a reactive way of looking at data management), with a focus that was often confined to specific departments. In 2022, most organizations are approaching data management differently. They’re aiming to be far more proactive — and to stay competitive, they have to be.
It’s less about simply “cleaning up” messy data, and more about creating meaningful, long-lasting, company-wide change that will continue to drive value and inform decision making in the future. In other words, it’s all about becoming data driven across the board.
Here’s an infographic that breaks down this change in mindset and some common challenges that are forcing companies to rethink the way they approach data:
Approaching data reactively and in silos is a way of the past. To keep up with the intense pace of change, constant innovation, and evolving customer expectations in 2022, a proactive, holistic, organization-wide strategy is required.
This change is positive on multiple levels. It’s not just good for staying competitive — it’s also a way to ensure that each of the common challenges described above (talent optimization, business insights, technical debt, etc.) get addressed so you can reap the benefits of becoming a data-driven organization.
That said, embarking on a large data transformation project can sometimes feel impossible, especially if you can’t promise ROI until months (or years) down the road. At The Gunter Group, we believe in taking a different, more iterative approach that enables organizations to realize immediate value while still keeping their larger goals — and the overall data landscape — in mind.
Ready to reframe the way your organization thinks about data? Talk to the experts at The Gunter Group.
What is tech debt?
Technical debt is often defined as the cost incurred when you repeatedly choose short-term solutions rather than doing the (larger, more expensive) work of tackling the big-picture causes of your problems.
But let’s look at the issue through a different lens: what is the nature of technical debt?
Because new solutions are built and deployed every day, all organizations incur tech debt, to some degree, with every system and process implementation decision they make. Even if you implement a new, innovative solution today, there will be a better one available tomorrow. In this scenario, you will still incur tech debt — just less than an organization that makes no updates.
Too many organizations think of tech debt as a problem that can be permanently solved. In reality, it’s a constant that’s renewed continuously by change and growth, and trying to “solve” it completely is a futile pursuit.
While you can’t make tech debt vanish into thin air, you can certainly make it more manageable. If you focus on managing its impacts in an ongoing way, you can deflate its looming, monstrous reputation and get to work on making meaningful improvements in the here and now.
Is tech debt destroying your data-driven dreams?
Analytics bottlenecks are a common issue related to tech debt. Silos slow down the analytics process; if only one person knows where a spreadsheet is and how to extract meaningful data from it, they become the bottleneck.
With each short-term fix and siloed process, data becomes harder to manage, access, and analyze. In turn, drawing insights from that data requires more time and effort, the insights become less timely and less reliable, and informed decision making becomes more challenging.
In other words, tech debt has a way of draining value from data — and the longer you let that debt accrue, the more value you’re losing. Using a prioritized approach to managing tech debt can help you cover more ground right out of the gate, so you don’t lose any more value than you have to.
One way to apply this prioritized approach is with backlog grooming, the periodic process of reviewing and prioritizing backlog tasks (and removing unnecessary or outdated tasks).
How do you prioritize what areas to address?
There is a lot of information available on how to tackle tech debt. Unfortunately, most of it is theoretical. While the abstract stuff can be valuable, if you’re looking for a practical way forward, you need to bring your considerations back down to earth and fold in the business perspective to create a technical debt prioritization plan.
You probably have a lot of tools at your disposal, internally and externally, and resources to leverage. Take a look at what you need to have happen — not theoretically (e.g. eliminating all technical debt by some point in the future) — but actually.
For example, The Gunter Group recently worked with a retail automotive company that was struggling with data debt. It was impacting every area of their business, including employee satisfaction, customer satisfaction, and profit margin. They needed a new approach, but with such a vast problem, it was difficult for them to know where to start.
We worked with multiple teams within the company, including manufacturing, HR, marketing, and technology innovation to create a prioritization plan. High priority initiatives included redefining company-wide KPIs, designing and implementing machine learning algorithms, and improving data literacy across departments.
Though they still have a long way to go on their data maturity journey, this company was able to start making changes where it mattered most, rather than remaining paralyzed by the challenge ahead.
How we work with clients to tackle tech debt
Remediating data-related tech debt requires far more than just technical skills — it requires asking the right questions, gaining a holistic understanding of your organization’s business goals (as well as how they may vary across different departments), and creating a dialogue to explore possible solutions.
Each of these components requires a tremendous amount of time, which internal teams rarely have. In most cases, managing ongoing operational struggles takes priority over transformation, and team members don’t have the capacity to focus all their energy on addressing tech debt. Meanwhile, recruiting new team members is a time-consuming, resource-intensive process, and thanks to the tech talent shortage, it’s more challenging than ever.
Turning to outside help can get the data transformation ball rolling without overwhelming internal teams or opening a can of recruitment worms.
At The Gunter Group, we leverage a multidisciplinary approach (technology, people, strategy, and execution) that enables us to see the long-term big picture while solving the highest-priority problems in the short term.
Combined with our extensive technical capabilities, this approach allows our clients to chip away at their technical debt and reclaim the value of their data as quickly as possible — without the burden of hiring a new team.
Conclusion
Think about a meaningful, specific problem you’re facing right now that’s rooted in technical debt, and what you would be able to accomplish if this problem was being managed proactively.
If you set your sights on eliminating tech debt across your entire organization, you’ll likely get caught up in a complex tangle of issues — and that one major problem that’s holding you back now will still be holding you back in six months.
To accelerate your progress, identify your most pressing issues, and reach out to expert help if you need it. With the right strategy and the right partner, you can mitigate tech debt and use your data to its fullest potential.
Is technical debt slowing you down? Discover how to improve your data infrastructure and decision making with workshops hosted by The Gunter Group.
ENGAGEMENT SPOTLIGHT
WITH SCOTT THEENER
Every organization we work with was deeply impacted by the COVID pandemic. Care for people, economics, health and safety: every company and organization has had to adapt to a very different set of priorities.
In the thick of the pandemic lockdowns, leading up to certain businesses opening back up, a multi-campus community college came to TGG asking if we could help solve a problem that had no pre-fab solution.
New state and federal health and safety protocols required the Auditing Team team to quickly react, building new campus regulations resulting in an exponential increase in procedural tracking, documentation, and compliance reporting.
I worked with our client to track the ever-changing regulations in order to develop a method to integrate the changes into scalable processes. The engagement was successful, and engaging for the whole team because there was a vision for the future.
To begin, I had introductory sessions individually with all team members to learn what was going well and what they felt could be improved. This helped inform a prioritized list of opportunities that we could start working on. We identified opportunities to implement automation and create recurring collaboration sessions where the team drove their own solutions to group challenges, rather than deferring only to management guidance.
TGG then supported this process by performing a gap analysis of the state and federal regulation changes and creating easy to consume documents for interested parties. These analysis documents took hundreds of pages of regulation and distilled them into language that increased awareness and increased understanding of the changes. This allowed staff and faculty to quickly create health and safety plans for their colleges that ensure compliance with the regulations while also creating safer environments.
I also facilitated multiple sessions with the team to ensure new regulations were accounted for in the organization’s processes and the team felt confident the processes could continue the work.
By design, my primary function in these sessions was to ask questions to the team, usually without knowing the answer. The intention was to utilize “the wisdom of the crowd”. This philosophy states that the collection of wisdom from the people doing the work is the best way to improve and solve that team’s challenges.
Another goal we established for this engagement was to help the team become more “T-Shaped”. Each team member had a specialty and expertise they brought to the team (the vertical bar of the T) while the horizontal bar of the T represents the knowledge they gain by learning about and exercises the expertise of their teammates. While being an expert in a particular discipline or field ensures the team has a wide range of skills, a teammate who is interested in learning new skills from their colleagues, helps to expand the shared understanding of the team as a whole. For example, one result of this effort and focus was that everyone on the team expanded their data analysis and spreadsheet skills.
The final piece of the project was helping the client to level-up and automate their data collection and analysis tools. Helping the client improve their data maturity and strategic data use, helped deliver efficiencies and insights that allowed the team to focus on areas that needed more attention.
As I reflect back on this engagement, the most rewarding component of the work was knowing that the team’s investment in becoming self-organized and deeply collaborative truly helped keep staff, faculty, and students at the campuses safe and healthy. We delivered real, tangible outcomes.
The community college is now well-positioned to continue monitoring for regulation compliance, via data analysis and metrics, to help departments enable health and safety best practices. We helped deliver a safer learning and working environment for students and faculty throughout the institution. The Health & Safety team has new found confidence in their collaboration skills, preparing systems and processes, and the tools needed to successfully complete a health and safety project of this magnitude.
When I look ahead to supporting other clients in the future, I’ll be able to use this experience to advocate for the power and opportunities that data analysis and metrics provide teams, the unlocking of potential when a team sees each other as equals and values collaborations with each other, and that communicating with your partners and stakeholders with transparency and honesty is truly a non-negotiable of success.
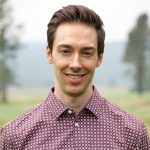
Scott has 10 years of dedicated experience as an Agile practitioner, Scrum Master, and Product Manager coaching teams, mentoring new Agilists, and leading successful projects. With his high attention to detail and a passion for independent research and translating findings into compelling visual presentations, Scott excels at change management, team facilitation, and emotional intelligence. He is known for being a natural detective and storyteller who is committed, organized, and a responsible teammate that prioritizes consensus and transparency in all situations. Scott holds a B.A. in Mass Communication/Journalism from Boise State University. He is also a Certified SAFe 5 Agilist, Advanced Scrum Master, Scrum Master, and Product Owner. Outside of work, Scott loves exploring Oregon’s great outdoors and is an avid college football fan who loves rooting for his Boise State Broncos. He also loves Portland’s independent theaters such as Laurelhurst Theater, Hollywood Theater, and Cinema 21.
REAL WORK. REAL RESULTS.
PRAGMATIC AGILE IN PRACTICE
We recently published a series of articles on the topic of pragmatic Agile and how its approach and methods can impact organizations of all sizes. As a way to highlight pragmatic Agile in real life practice, we wanted to provide an example of how one of our consultants utilized pragmatic Agile while supporting a client.
Senior Consultant Rob Anteau, has been working with Agile and waterfall teams for decades. Even before joining TGG, he developed a similar perspective to the implementation of Agile. Below is a recent example of an impactful Agile adoption Rob oversaw at one of our clients.
Rob was a project manager leading an effort to modernize a software platform used by the client. The project was sponsored by “traditional IT” and many leaders dismissed Agile concepts in their initial plans, nor was that even part of their culture. Rob challenged this.
First, Rob left the Agile vocab and dogma at the door. The company culture wasn’t hospitable to the new terminology, so he didn’t push it. Rob started with a two week time-box with a planning session. The team got on board, finding freedom in the admission that they didn’t know everything at the beginning of the project.
Next, Rob introduced a retrospective, tailored specifically to his team. He framed it as a chance for the team to learn from their mistakes and to capitalize on strengths. He led by example, demonstrating what active engagement looked like.
This all required some heavy lifting on Rob’s part. He still had to create the 650 line project plan, and constantly translated the iterative work of his team into a report for leadership. He served as a lead blocker, allowing his team to iterate while he kept management informed. In the end, all parties were happy with the new setup.
Rob knew that elements of Agile would be helpful for his team, and understood he didn’t need to get there in one day. He took his time, gradually introducing elements and demonstrating their value. He didn’t need to act like the smartest guy in the room; rather, through servant leadership he demonstrated and cultivated the Agile mindset. As a result, his team experienced a mindset-shift, finding comfort in the idea that their work didn’t need to be perfect to be valuable. They came to see “good enough for now” as, well… good.
This was pragmatic Agile in practice. Whether our clients need support for a wholesale digital Agile transformation or just a little help along the way, we’re excited to partner with them to meet their goals.

More about Rob Anteau:
Rob is a technical program leader who is adept at developing and executing programs utilizing agile and waterfall methodologies across multiple industries, from healthcare to the public sector. With a background in IT infrastructure, cloud migrations, network operations, and cyber security projects, Rob uses his technical expertise and business acumen to bring stakeholders together to ensure quality and timely delivery. He places importance on communication and being adaptable to a variety of environments. Understanding that there is no one-size-fits-all solution in technology, Rob is committed to delivering a final product that is aligned with client objectives. Rob holds a B.A. in Industrial and Organizational Psychology from Vrije Universiteit as well as the following certifications; SAFe 5 Agilist, Scrum Master, ITIL, ISTQB, Prince2, Six Sigma Green Belt, and TMap. Rob is a fan of the maker movement and in particular enjoys electronics projects. His other passion is anything VW related; he owns a 1978 Westfalia.